16th Conference on Computer and Robot Vision, 2019
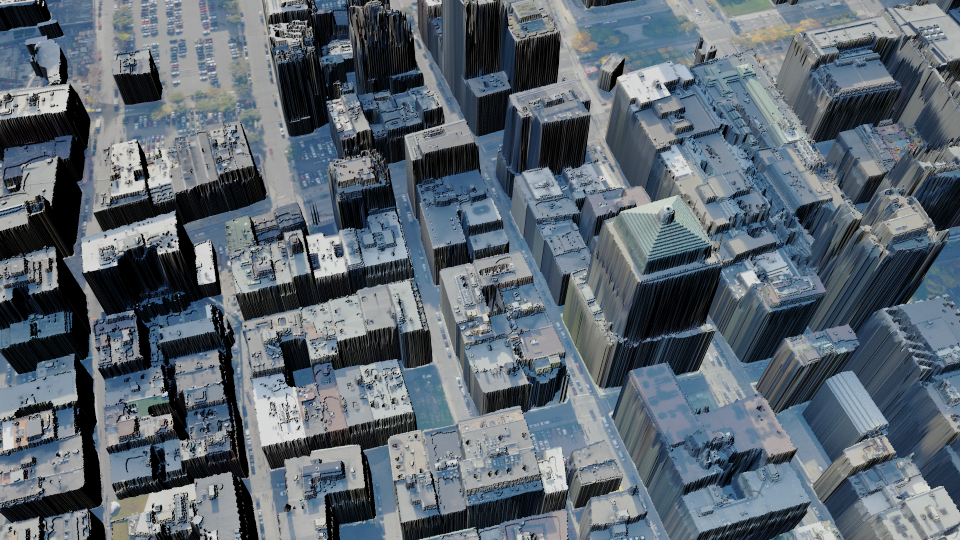
Join us at the 16th Conference on Computer and Robot Vision, 2019
ICT lab researcher Bodhiswatta Chatterjee will be presenting our work “On Building Classification from Remote Sensor Imagery Using Deep Neural Networks and the Relation Between Classification and Reconstruction Accuracy Using Border Localization as Proxy”. The work is co-authored with Bodhiswatta Chatterjee and Charalambos Poullis.
Abstract: Convolutional neural networks have been shown to have a very high accuracy when applied to certain visual tasks and in particular semantic segmentation. In this paper we address the problem of semantic segmentation of buildings from remote sensor imagery. We present ICT-Net: a novel network with the underlying architecture of a fully convolutional network, infused with feature re-calibrated Dense blocks at each layer. Uniquely, the proposed network combines the localization accuracy and use of context of the U-Net network architecture, the compact internal representations and reduced feature redundancy of the Dense blocks, and the dynamic channel-wise feature re-weighting of the Squeeze-and-Excitation(SE) blocks. The proposed network has been tested on INRIA’s benchmark dataset and is shown to outperform all other state-of-the-art by more than 1.5% on the Jaccard index.
Furthermore, as the building classification is typically the first step of the reconstruction process, in the latter part of the paper we investigate the relationship of the classification accuracy to the reconstruction accuracy. A comparative quantitative analysis of reconstruction accuracies corresponding to different classification accuracies confirms the strong correlation between the two. We present the results which show a consistent and considerable reduction in the reconstruction accuracy. The source code and supplemental material is publicly available at https://www.theICTlab.org/lp/2019ICTNet/.